Customization is a ubiquitous part of modern life. But have you ever seen customization applied to large-scale AI models? š¤
While the customization of text-to-image models is rapidly advancing, the customization of text-to-video models remains in the research stage. Google DeepMind’s groundbreaking Still-Moving framework has now achieved customized generation for T2V models!
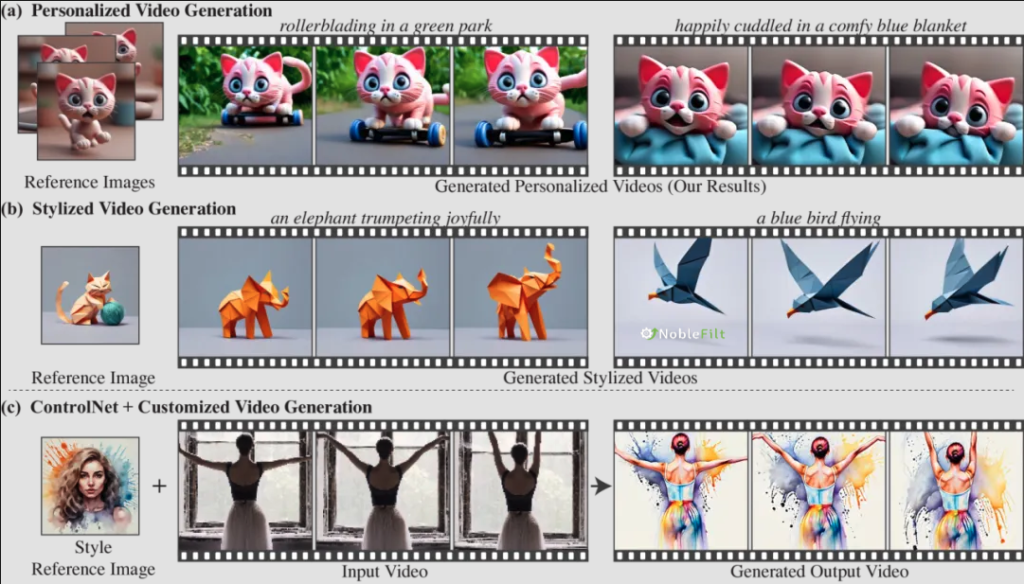
Project Overview: Enabling Customized Video Generation
The primary obstacle to video generation customization has been the scarcity of customized video data.
Still-Moving is an innovative, general-purpose framework that enables the customization of text-to-video models without necessitating customized video data.
Given a T2V model built upon a T2I model, Still-Moving can align any custom T2I weights with the T2V model using only a small set of static reference images, all while preserving the T2V model’s motion priors.
Impressive Demos: Personalized and Stylized Video Generation
Below are examples of personalized video generation achieved by adjusting personalized T2I models:
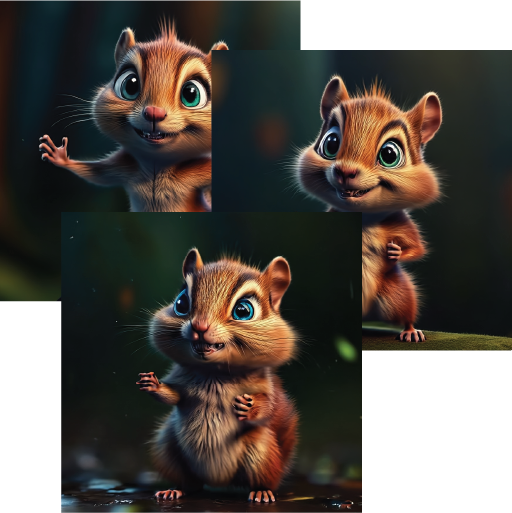
Still-Moving can also generate stylistically consistent videos based on pre-trained stylized T2I models.
The following examples showcase videos that adhere to the style of the reference images while exhibiting the natural motion of the T2V model:
Key Principles: Harnessing Motion Priors
When presented with a set of static images, we can readily envision the dynamic changes of the subjects under various scenarios. š¾
This ability arises from our robust prior knowledge of object motion, physics, and dynamics.
The core question driving this research is: Can a generative video model that has learned motion priors be leveraged to achieve human-like imagination capabilities? š¤
Still-Moving proposes a method that directly extends the customization results of T2I models to T2V models, eliminating the need for customized video data.
A Two-Step Customization Process
Still-Moving achieves customization through a two-step process:
- Motion Adapter Training: Motion adapters are introduced to control the amount of motion generated by the model in videos. By training these adapters on static videos, the model learns to generate static videos.
- Spatial Adapter Training: Customized T2I weights are injected, and spatial adapters are trained on data that combines customized images and natural videos. This allows the model to adapt to customized spatial priors while maintaining its motion priors.
The team demonstrates the effects of using motion adapters at varying ratios.
Proven Effectiveness Across Multiple Tasks
The DeepMind team has demonstrated the effectiveness of the Still-Moving framework across multiple tasks, including personalized generation, stylized generation, and conditional generation.
In all evaluated scenarios, Still-Moving successfully combines the spatial priors of the customized T2I model with the motion priors of the T2V model to generate high-quality video content.
Applying Still-Moving to the AnimateDiff T2V model and comparing it with simple injection, the second row showcases the superior results of Still-Moving.
The team also conducts a qualitative comparison between Still-Moving and baseline methods. The last column highlights the impressive effects achieved by Still-Moving.
Conclusion: Expanding T2I Customization to Video Generation
Still-Moving expands the customization results of T2I models to the realm of video generation, addressing the key challenge posed by the lack of customized video data.
The DeepMind team’s innovation has unlocked the potential for high-quality customized video generation. We eagerly anticipate the team’s future contributions to the rapidly evolving field of AI generation!
š Project Link: https://still-moving.github.io